DeSci + AI: Say Goodbye to Tedious Literature Reviews!
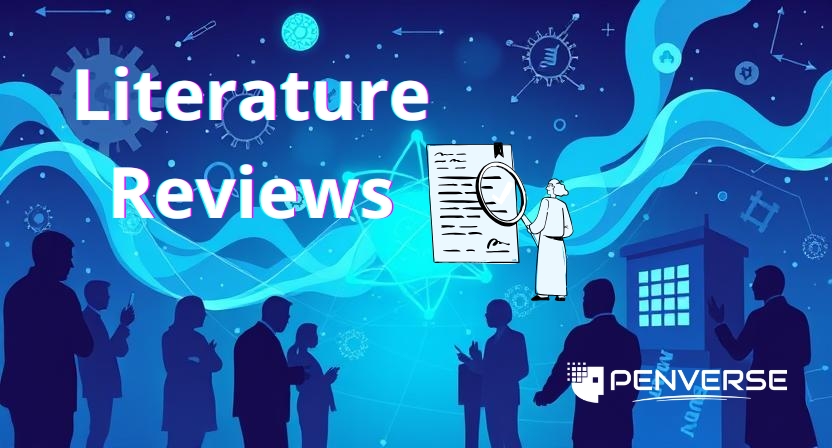
The Burden of Traditional Literature Reviews
Conducting literature reviews is a fundamental step in the research process, yet it is often fraught with challenges that can hinder progress. Researchers are required to meticulously search, analyze, and synthesize vast amounts of academic literature before initiating their own studies. However, this process is not only time-intensive but also prone to errors and biases that can affect the overall quality of the research.
A significant issue with traditional literature reviews is the sheer volume of published research. Every year, millions of new research papers are published across various disciplines, making it difficult for researchers to keep up with the latest advancements. This information overload forces scientists to either spend months reviewing existing literature or risk missing critical findings that could impact their work. Additionally, traditional reviews depend on manual efforts, where researchers must filter through thousands of articles, often using outdated search methods that fail to capture the most relevant studies.
Beyond the excessive time commitment, bias in literature reviews is another problem. Researchers may unknowingly favor studies that support their hypotheses while disregarding conflicting findings. Such biases can lead to flawed conclusions and reduce the credibility of research outcomes. Furthermore, reproducibility remains a challenge, as different researchers may adopt different search strategies, leading to inconsistencies in findings. A study highlighted that traditional reviews often lack appropriate critical appraisal, treating all evidence as equally valid, which can compromise the quality of the review (LSE Blog).
The Emergence of AI in Literature Reviews
Artificial Intelligence (AI) is revolutionizing the way literature reviews are conducted by automating various stages of the process. With AI-powered tools, researchers can complete literature reviews in a fraction of the time, without compromising quality or accuracy. These AI systems utilize advanced natural language processing (NLP) algorithms that can read, understand, and extract relevant data from vast repositories of academic papers.
One of the most impactful AI capabilities is automated screening. AI-powered research assistants can rapidly scan and filter thousands of academic papers, identifying the most relevant studies based on keywords, citations, and topic relevance. This eliminates the need for manual sorting and ensures that only the most crucial research is considered.
Another important aspect of AI integration is data extraction. AI-driven tools can extract essential information from studies, such as key findings, methodologies, and statistical results. This allows researchers to quickly compile and compare different studies, identifying patterns and trends that would be challenging to detect manually.
Quality assessment is also enhanced through AI. Unlike human reviewers who may overlook errors or inconsistencies, AI-powered systems can evaluate the credibility, reproducibility, and statistical validity of studies before including them in a review. For instance, AI has been applied to automate the literature screening procedure in medical systematic reviews, enhancing efficiency and accuracy (Systematic Reviews Journal).
Statistics Highlighting AI's Impact
The integration of AI into literature reviews has yielded significant improvements across multiple dimensions:
- Efficiency Gains: AI-assisted screening can reduce the time required for literature reviews by up to 50% (John Snow Labs).
- Improved Accuracy: AI tools have demonstrated higher accuracy in identifying relevant studies compared to manual methods, reducing human errors.
- Cost Reduction: Automating literature reviews leads to substantial cost savings, as researchers spend less time on preliminary research and more time conducting impactful experiments.
- Comprehensive Coverage: AI ensures that no relevant research is overlooked, unlike human reviewers who may unintentionally skip studies due to information overload.
These statistics demonstrate that AI is not just an enhancement—it is a fundamental shift in how scientific literature is reviewed and utilized.
Penverse: Harnessing AI for Superior Literature Reviews
Penverse, a pioneering platform in Decentralized Science (DeSci), is at the forefront of integrating AI-driven research methodologies. By leveraging AI-powered literature review agents, Penverse is transforming the way researchers engage with scientific literature, ensuring greater accuracy, efficiency, and transparency.
AI Research Agents for Automated Literature Review
Penverse employs autonomous AI research agents capable of performing comprehensive literature searches, analyzing existing studies, and summarizing key insights. These agents utilize machine learning algorithms to prioritize high-quality research, eliminating bias and ensuring that researchers have access to the most relevant findings.
Unlike traditional literature review methods that require researchers to spend weeks manually sorting through papers, Penverse’s AI-driven system can complete a thorough literature review in hours. This rapid turnaround accelerates research timelines and allows scientists to focus on experimentation and discovery rather than administrative tasks.
Decentralized Collaboration for Transparent Research
One of the core principles of Decentralized Science (DeSci) is open collaboration. Penverse integrates blockchain technology to ensure transparency in literature reviews. By storing literature review records on an immutable ledger, Penverse allows researchers to verify the sources, methodologies, and selection criteria used in AI-driven reviews. This prevents manipulation and enhances trust in the scientific community.
Furthermore, Penverse’s decentralized model allows researchers from different disciplines and geographic locations to collaborate in real time. Through a shared AI-powered knowledge graph, scientists can identify overlapping research areas, reducing redundant efforts and fostering cross-disciplinary innovation.
Continuous Learning for Enhanced Literature Reviews
Unlike static literature review models, AI agents in Penverse are continuously learning from new research papers, citations, and user interactions. This ensures that literature reviews remain up-to-date with the latest findings and emerging trends. The ability of AI to learn and improve over time enhances the accuracy and relevance of literature reviews, ensuring that researchers are always working with the most current and credible data.
Conclusion
The integration of AI into literature reviews is eliminating traditional bottlenecks, allowing researchers to save time, reduce errors, and improve the quality of their work. By leveraging AI-powered research assistants, scientists can conduct more comprehensive and unbiased literature reviews, leading to stronger and more reproducible research outcomes.
Platforms like Penverse are leading this revolution by combining AI, blockchain, and decentralized collaboration to create a transparent and efficient research ecosystem. By embracing AI-powered literature review systems, researchers can ensure faster, fairer, and more accurate scientific progress.
🚀 Say goodbye to tedious literature reviews—embrace the AI-powered future with Penverse today! 🚀